Let’s Make Sure the Right-Hand Rule is Left-Behind
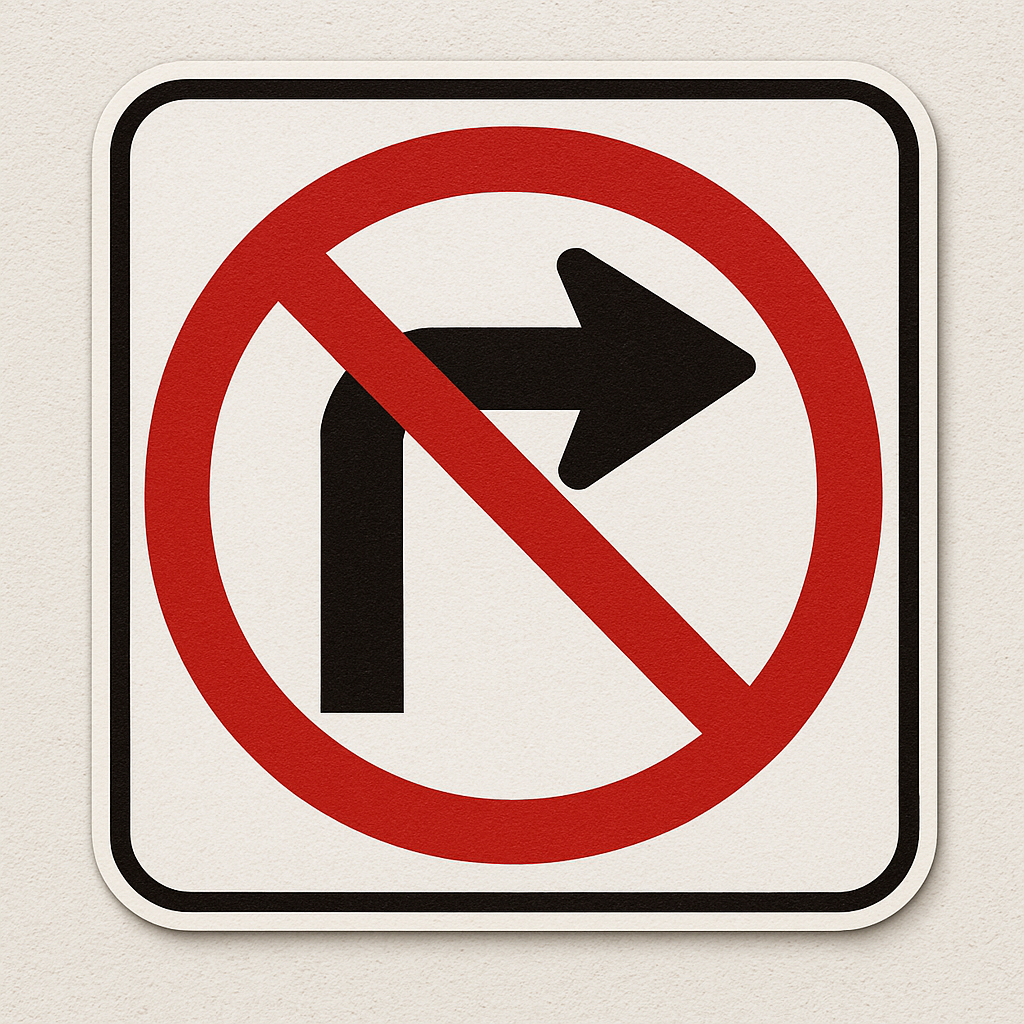
Household surveys are a critical source of data for understanding the conditions, experiences and aspirations of families. Governments and social sector organizations use data from household surveys to inform program design, targeting, service delivery, budget allocations and more. Household surveys give families a voice – through data – in the policies and programs that affect their lives. But it is impossible to reach all households, and not all households are alike. So how do surveyors choose who to visit to ensure that their data are representative of the wide variety of families in a given place? That is a question about sampling.
Ideally (at least from a survey perspective), we would have a list of all households from which we could randomly select a subset for surveying. Unless you are conducting research in a select few Nordic countries, you are unlikely to have access to such a list. In the absence of a household list, rigorous surveys like the Demographic and Household Survey and Living Standards Measurement Survey typically sample households by selecting areas, conducting a complete listing of all households in each selected area, and then randomly sampling households from these lists. This approach ensures unbiased estimates but is costly, tedious and time-consuming. The cost and time to get high-quality samples is a major impediment to more widespread use of timely, representative household data to inform programs and policies.
When trying to save time and money, a more common approach is to use the “right-hand rule” to select households within selected areas. With the right hand-rule, researchers still first select areas but, within each area, use a pseudo-random procedure (described below) to select households. Compared to the conventional approach described above the right-hand rule is very affordable. But is it reliable?
As part of DataDelta’s research initiative on innovative household sampling techniques and ongoing work to strike a better balance between quality and cost in household surveys, we tested the right-hand rule. We found that the right-hand rule is far from reliable. It suffers from three concerns that can jeopardise both the representation and quality of the resulting data. In our research, the right-hand rule:
- Excludes a large portion of households;
- Oversamples some households and undersamples others, making it likely that resulting data will not truly represent the population; and
- Is nearly impossible to implement with any consistency, hindering the ability to verify compliance with the sampling protocol or to verify data quality through standard backchecks
In cases where researchers don’t have the budget to conduct a complete household listing (or this is infeasible due to lack of granular census data), we recommend a new alternative pioneered by the DataDelta research team: rooftop sampling. We belief rooftop sampling strikes a much better balance between quality and cost. (See the last section for links to more information.)
How the right-hand rule works
To our knowledge, there is no definitive description of the right-hand rule online. Based on our best understanding (compiled from various written materials and conversations with field managers who have used the right-hand rule on previous projects), the steps to use the right-hand rule to sample households in primary stage units are as follows.
- Select areas, or primary stage units, (same first step as other sampling methods) and within each primary stage unit:
- Divide up the primary stage unit into four sub-areas
- Within each sub-area pick:
- Pick a central starting point
- Start at the starting point and start walking, taking right-hand turns whenever possible
- Survey every 5th household on the right-hand side of the road until you have surveyed 5 households (for a total of 20 households in the entire village)
The diagram below provides a graphical representation of how the right-hand rule works.
Problem 1: A lot of households don’t live on a road
Since surveyors select households by walking along roads, the right-hand rule excludes households that don’t lie on any road. To estimate the share of structures that don’t fall on a road in the Philippines, we randomly sampled .01% of all rooftops (1189 total rooftops) in the Philippines from the Google Open Buildings dataset and then used the Google “snap to road” API to determine whether the rooftop was close to a road. For all rooftops that the Google API indicated was not on a road, we conducted a visual inspection of Google Maps satellite imagery to double-check that: a) the rooftop was indeed a rooftop of a building that appeared to be inhabited; and b) the building was indeed far enough from any road that a surveyor would likely not select it using the right-hand rule. We found that 16.7% of all rooftops in the Philippines don’t lie on a road. The image below shows one example of a sampled rooftop not near a road:
This doesn’t necessarily mean that 16.7% of all Filipino households would be excluded using right-hand rule sampling since it doesn’t take into account how many people live in each structure. (Intuitively, we would expect multi-household residential structures to be more likely to fall on a road. On the other hand, commercial structures would also be more likely to fall on a road.) And results for other countries may obviously be different. Still, it suggests that the right-hand rule likely systematically excludes a lot of households, especially in very rural areas and slums, likely biasing the results.
Problem 2: It over-samples some households and under-samples others
Ideally, the right-hand rule would assign equal weight to all households in a sampled area. Yet the right-hand rule over-samples some households and under-samples others. First, the right-hand rule over-samples households in sparsely populated areas and under-samples households in densely populated areas. To see why this is the case, imagine a simplified version of the right-hand rule where we pick a random starting point and direction, follow the road in the chosen direction (taking right turns where possible), and only survey the first household on our right. Then a given house will be sampled if and only if there are no other houses between the starting point and that house in the chosen direction while following the right hand rule. In the diagram below, the green line shows the set of all possible starting points that would lead to the green house being selected and the orange line shows the set of all possible starting points that would lead to the orange house being selected using this simplified version of the right-hand rule. Thus, if we were to randomly pick a point with equal probability, the green house would have a much higher probability of selection.
With the full version of the right-hand rule, things are a bit more complicated but the basic logic is the same: houses in densely populated areas have much lower probability of selection than houses in sparsely populated areas. The above discussion is just theoretical but the example image from the Philippines below shows that in most areas there is a mix of densely populated and sparsely populated areas.
Second, the right-hand rule under-samples households that live on the inside of a closed loop defined by the road network. For households that live on the inside of loops, the only way that they can be selected is if the chosen start point is inside the loop. The diagram below illustrates this and the examples from the Philippines underneath show that such instances are not that rare. (We estimate that approximately 2-5% of households in the Philippines lie on the inside of such loops.)
Problem 3: It is nearly impossible to replicate sampling results
The third, and most serious, problem with the right-hand rule is that it makes it nearly impossible to replicate sampling results. In other words, two surveyors given the exact same right-hand rule protocol and the same starting point and direction will typically survey completely different households. Replicability is crucial in sampling because it allows managers to check that surveyors have implemented the sampling protocol correctly. If a method can’t be replicated, managers have no way of checking that surveyors have implemented the protocol correctly. In-person data collection is difficult work. Even with good training, surveyors tend to survey the most easily available households or those which take the least amount of time to survey. As one field manager put it, if you have to ask detailed questions to each household member and you are unsure whether, according to the protocol, you should go to a household with a single elderly man or a household with 15 members, you will tend to choose the first household to save time. One goal of sampling protocols is to eliminate, or at least significantly reduce, enumerator discretion about which households to survey. The right-hand rule leaves too much to discretion.
We tested the replicability of the right-hand rule in extensive field tests in Uttar Pradesh in India. We trained 3 different experienced surveyors on the right-hand rule, randomly selected several different starting points and directions, and asked each surveyor to independently implement the right-hand rule from the same starting point and direction. The figure below shows the paths that the three surveyors took for one sample starting point. Each colored line represents a different surveyor and the numbered points represent households (with 0 representing the starting point.) As the figure demonstrates, the three surveyors went in nearly completely different directions.
The reason for so much variation among enumerators, even with the same protocol, is that there are many, many sources of ambiguity when you try to implement the right-hand rule in an actual real location. One very common source of ambiguity was whether a path or open space qualified as a road. For example, in the figure below, surveyors were unclear whether the open space marked by the red arrow was a road or just an open space. Another common source of ambiguity was whether a structure lay on the road that the surveyor was walking down or another road. In many cases, a surveyor could see a structure from the road he or she was walking on but the front entrance to the structure appeared, from the surveyor’s perspective, to lie on a different road. A third common source of ambiguity was whether a road had ended (and thus the surveyor should turn around).
In each of these cases, we tried to reduce the scope for ambiguity by adding increasingly complicated rules to our right-hand rule protocol. For example, if surveyors were unsure of whether an open space or alley should be treated as a road we instructed them to walk 10 meters down the open space / alley, check if there were any structures with an entrance facing the open space / alley, and, if there were, consider the open space / alley a road. Yet for every clarification we added complexity and a new source of ambiguity arose.
How to strike a better balance between cost and quality in sampling
The gold standard approach to sampling – including doing a full household listing, scores well on quality and representation but can be time- and cost-prohibitive. The right-hand rule scores well on cost but, as the three problems above suggest, it does not deliver the level of representation or quality needed to inform high-stakes decisions like where to target social service programs, or to accurately represent the conditions, experiences and aspirations of a diverse population. So what should researchers with high standards and a tight budget do? The DataDelta team at IDinsight recommends using rooftop sampling. It’s just as cheap and easy as the right-hand rule (if not cheaper and definitely easier to replicate) and, as we show in our forthcoming paper, much more likely to result in unbiased results.
We are on a constant quest to make it easier and more affordable to get high-quality, representative data directly from people so their voices and experiences can influence the policies and programs that affect them. Stay tuned for more on our cost effective approaches to rooftop sampling, data quality, and questionnaire development.